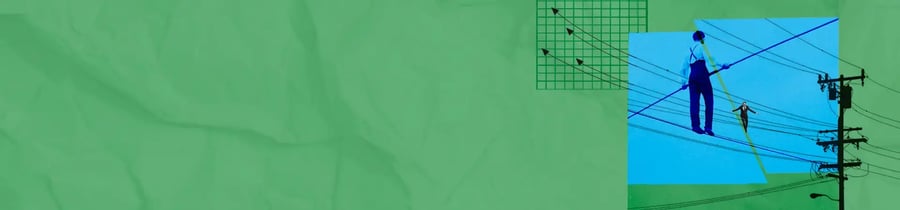
ITONICS INNOVATION BLOG
Let's talk innovation
Inspiring insights and best practices on innovation management, foresight, and strategy.
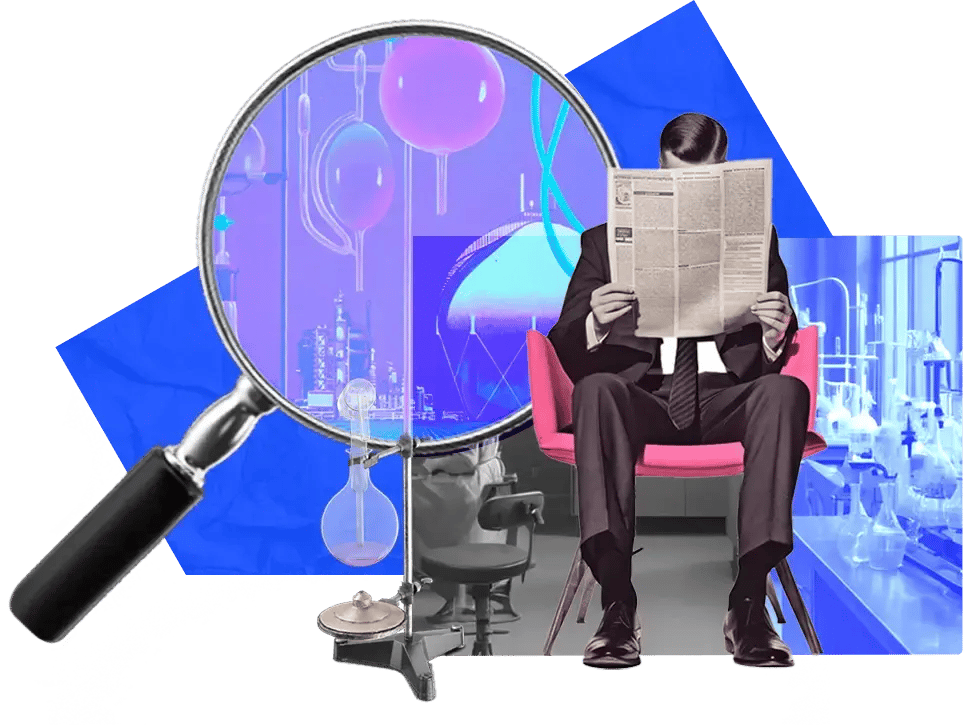
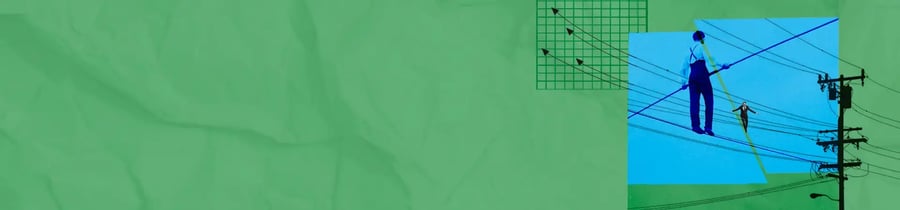
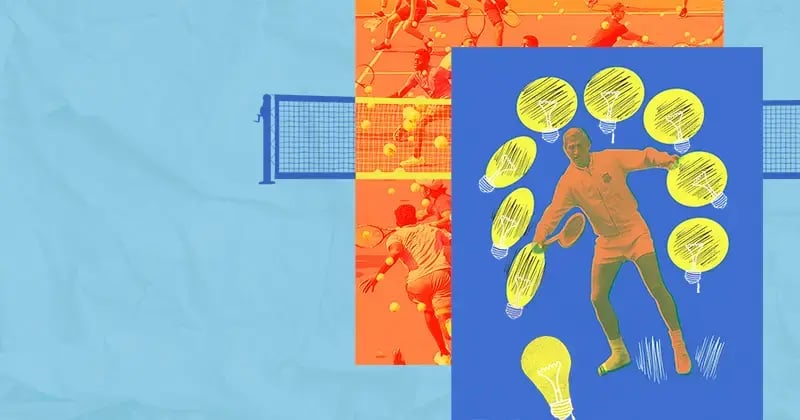
Bright Ideas In Your Suggestion Box: How to Boost Workplace Innovation
05 May 25 | 13 mins read
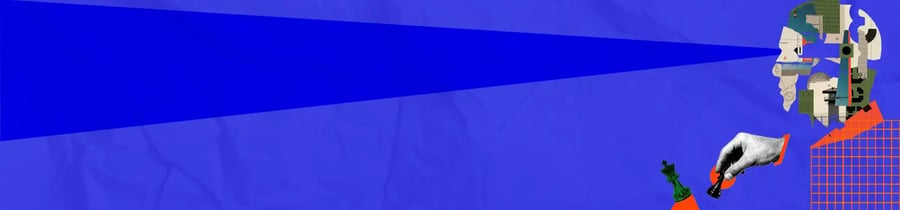
Chief Innovation Officers: Responsibilities, Strategies & Tools
29 Apr 25 | 8 mins read
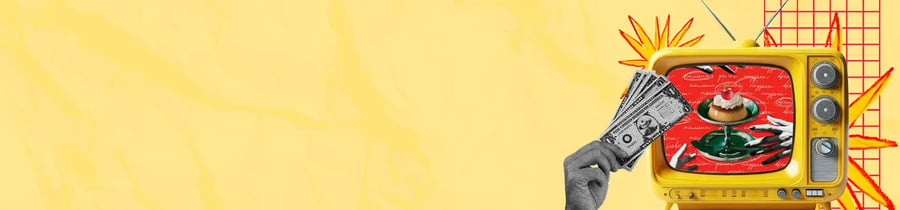
How to Develop New Product Ideas: 8 Proven Strategies
24 Apr 25 | 18 mins read
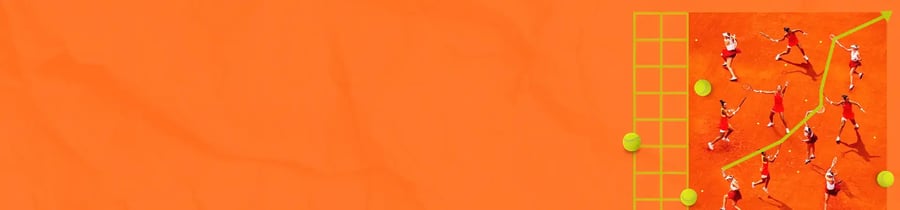
10 Process Improvement Hacks to Bring Bright Ideas to Market Faster
16 Apr 25 | 16 mins read
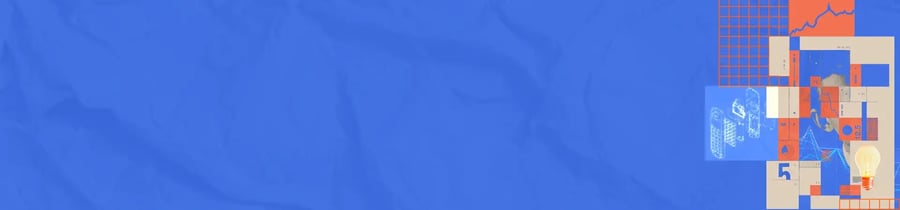
How to Run a Successful Hackathon: From Idea Generation to Real-World Prototyping
15 Apr 25 | 11 mins read
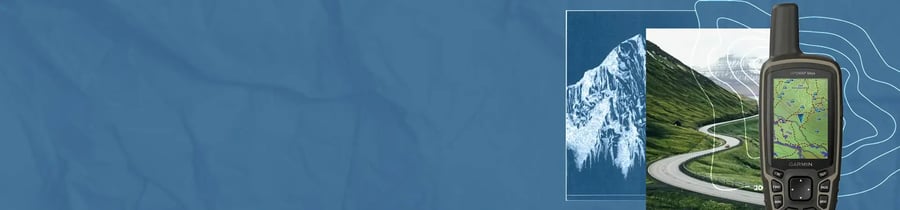
Top 10 Steps for a Clear and Future-Ready Technology Roadmap
07 Apr 25 | 10 mins read
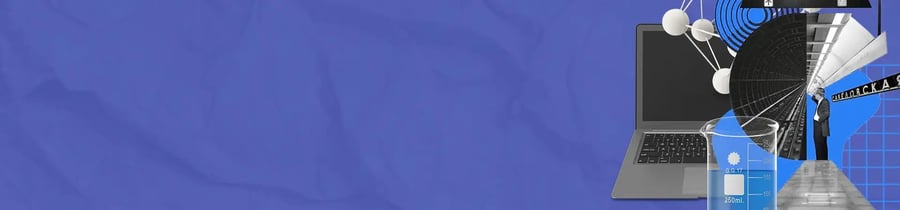
Best 6 R&D Management Software to Streamline R&D Operations
03 Apr 25 | 10 mins read
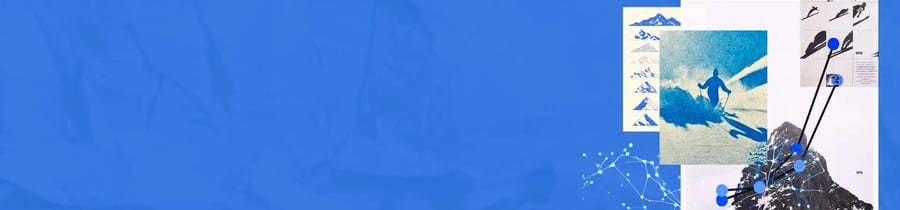
Impactful Innovation Intelligence: A Guide to Data-Driven Innovation
03 Apr 25 | 7 mins read